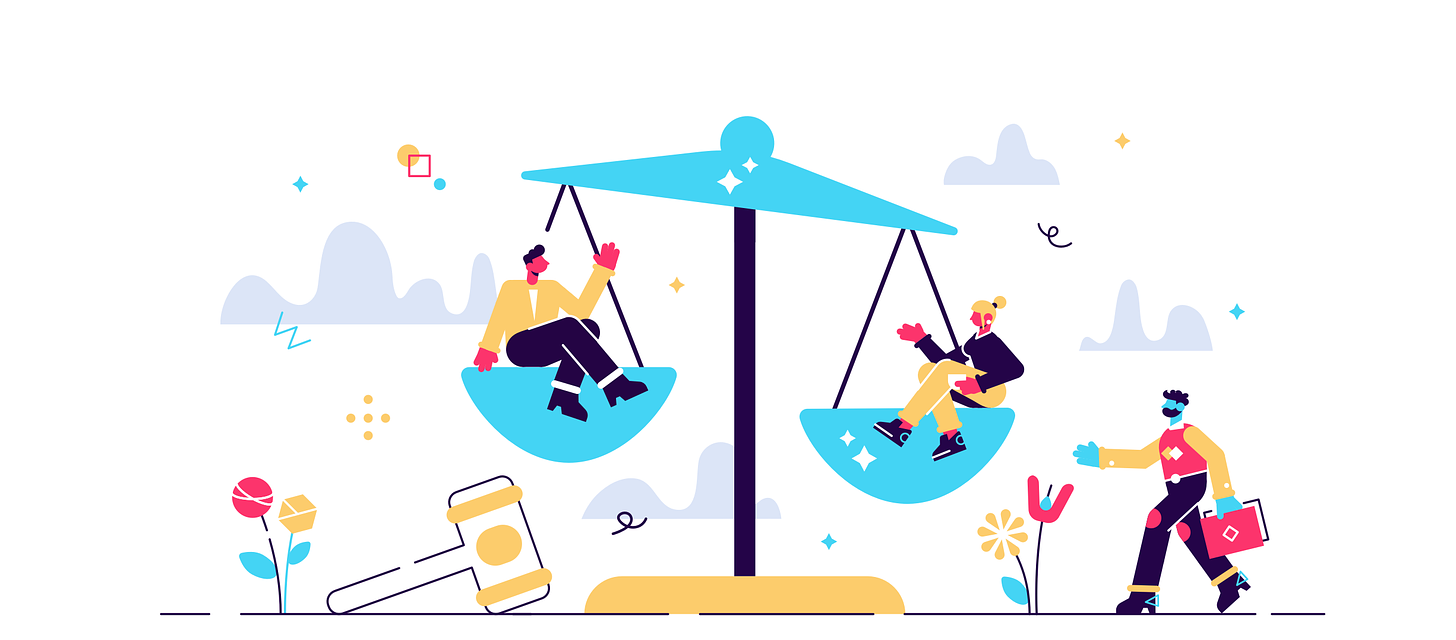
Benchmarking exists to make comparisons easier and help customers make an informed decision. There are benchmarks for CPUs, batteries, GPUs, shoe sizes.
Long term investments, like mutual funds or thematic (basket) investing, benchmarking a straight forward problem. Compare the performance to an index such as NIFTY 50, NIFTY 500, SENSEX or countless other indices.
To calculate how the investment is doing, closing price at the end of the trading day is checked. For past 1-year performance — look at the closing prices 1 year ago and today to calculate percentage change. This percentage change of the index and basket of stocks is compared to declare if the investment was not successful or not.
A similar exercise can be done for any period or frequency — from a day to many decades. Data for such benchmarking is readily available.
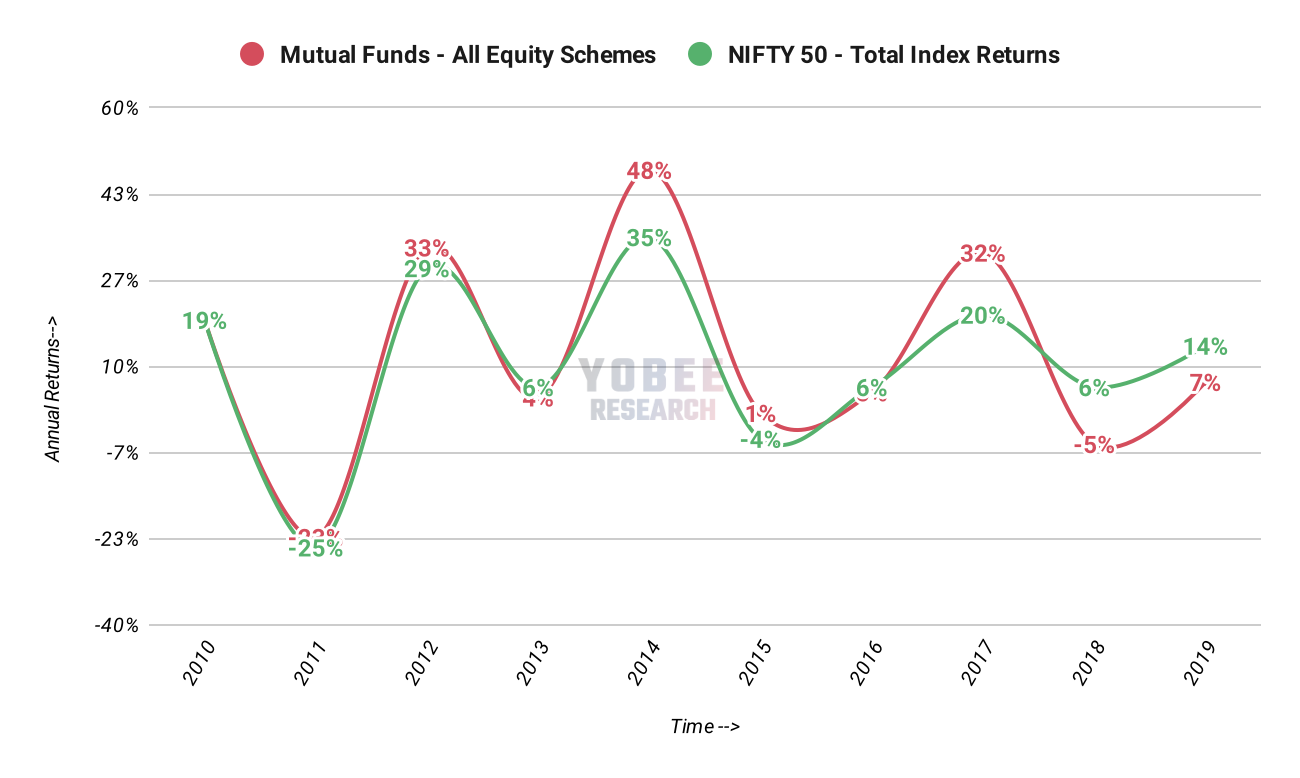
Commonly seen benchmarking graphs.
An often over-looked assumption is that the initial investment was not withdrawn. Therefore any gains/losses that happen in after/pre-market hours are automatically included.
An example — RELIANCE
- Closed at Rs. 1952.60 on 26th November 2020
- Opened at Rs. 1940.50 on 27th November 2020
- Closed at Rs. 1929.80 on 27th November 2020
This price difference of Rs. -12 between the close price on 26th and the open price on 27th is called gap-down. Gap-up is when the opening price is higher than the closing price of the previous day.
While calculating 1-day returns, just the closing prices on 26th and 27th is used, and the gap-up/gap-down is baked into it.
Quant strategies, especially intraday trading, take positions during the day and exited before the markets close. So these gap up/downs are not captured.
An obvious solution is to not calculate returns by taking close price between two dates — but instead calculate percentage change between the open to close price of each day and then compound all of them to get overall returns.
Let’s see how NIFTY50 performance would look like.
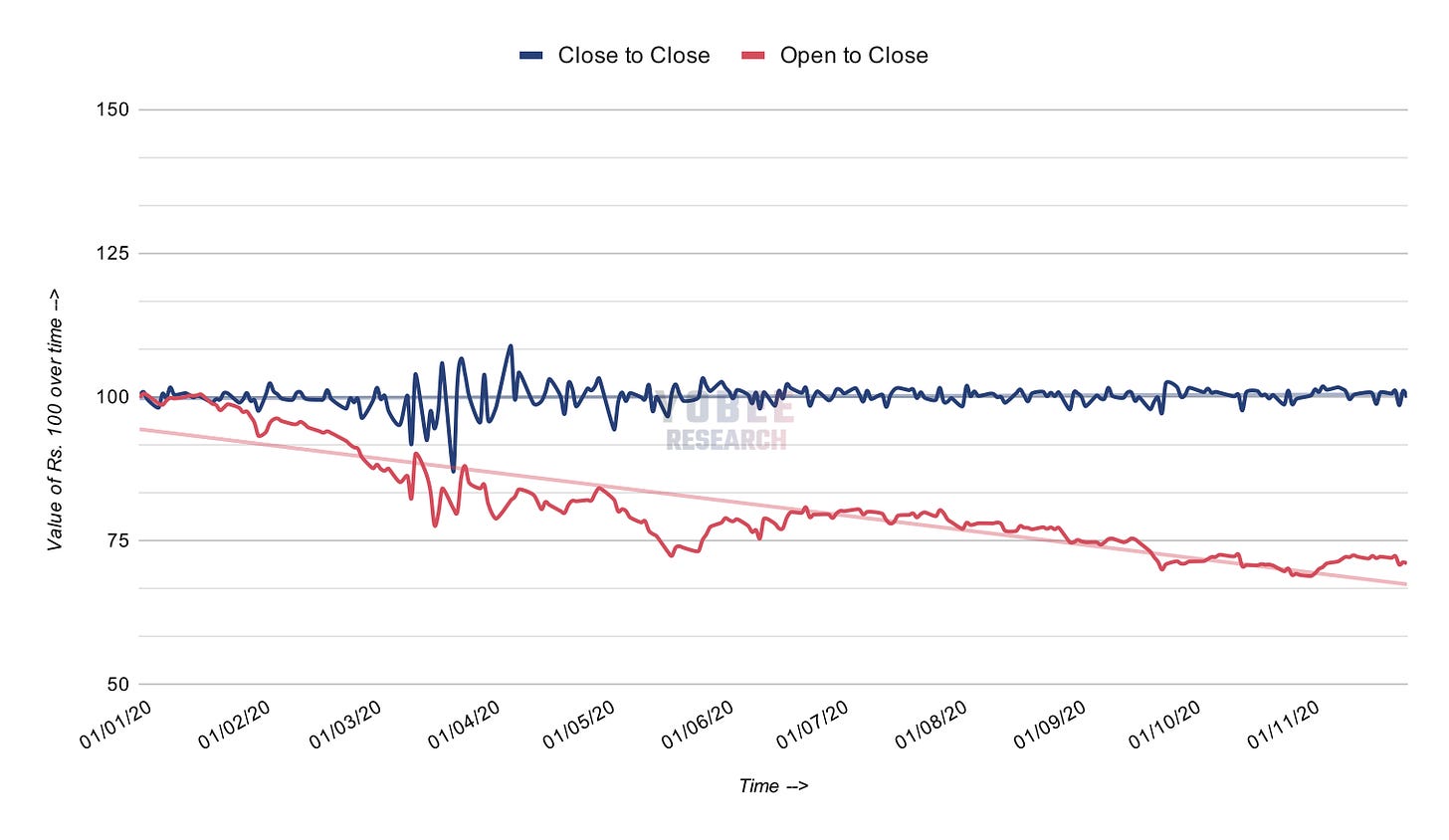
NIFTY 50 Performance in 2020
Close to close price returns till 28th November 2020 was -0.14%. Open to close price returns is -29.05%.
Astounding. The difference in final returns is 28.91%. In other words, the gap up/down contribution was 28.91%.
However, there’s a problem with this approach. In our open to close calculation, we buy at the open price and sell at the close price. In intraday trading, it’s possible to sell first (short sell) — sell at the open price and buy at the close price — flipping the calculation.
Let’s see graphs with the flipped assumption.
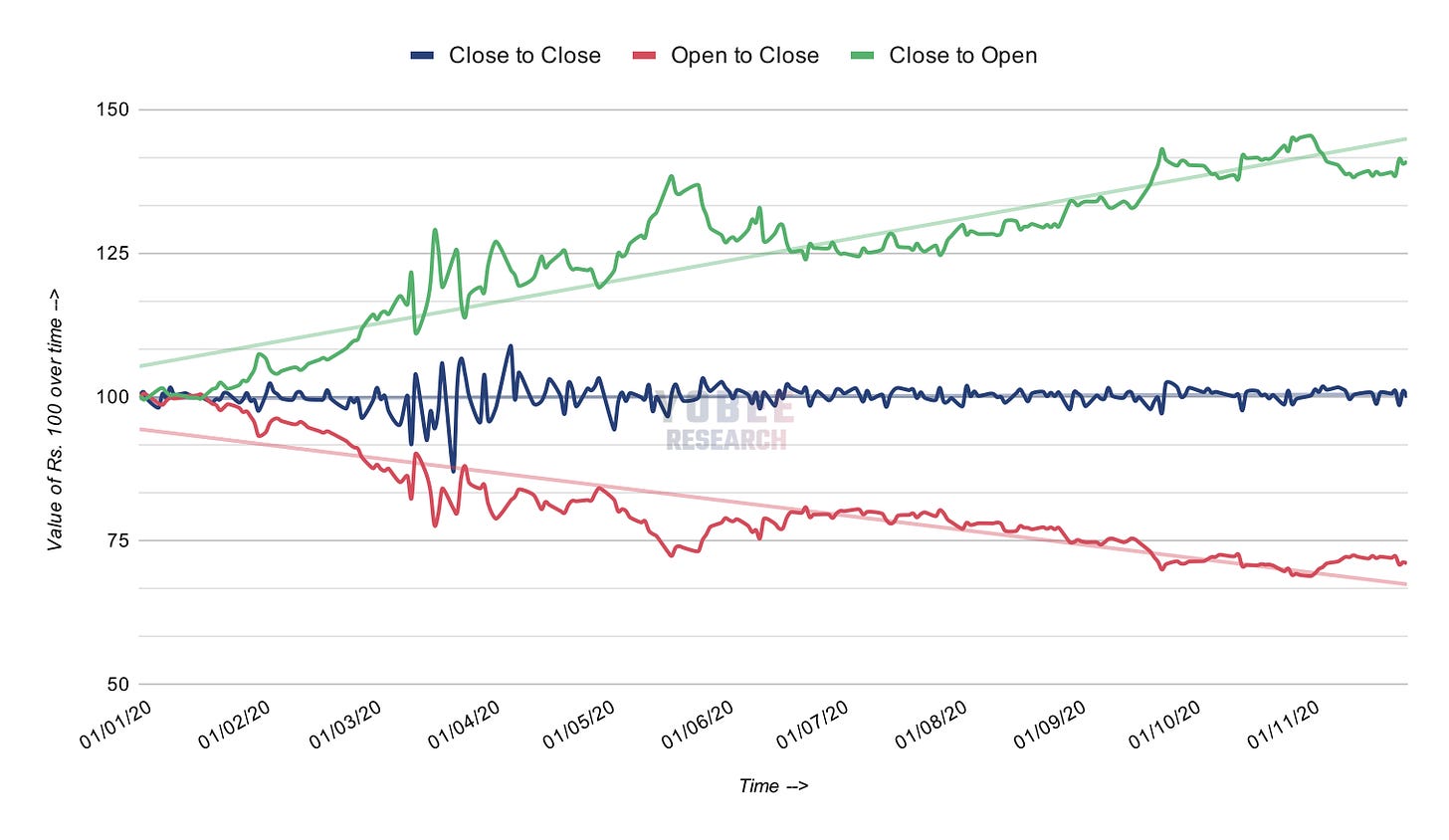
NIFTY50 Performance in 2020 with flipped returns
Close to open price returns are almost a mirror image of open to close price returns, which is to be expected.
Arbitrarily choosing to buy or sell first and computing returns is not a benchmark. It can easily be manipulated.
To fix this problem, a random decision on whether to buy or sell first each day is unbiased—the correct approach, when random sampling, is to apply Monte Carlo simulations. A random decision is made at least 250 times each day (number of trading days in a year) and then averaged. Some astute readers would have immediately uncovered a flaw — daily average returns would be close to 0%. That’s, again, not useful for benchmarking.
Does this mean that market indices can’t be used? It’s still debatable.
A promising solution to explore is benchmarking with other quant trading strategies.
Some institutions track quant funds across the globe. Two of them are — Hedge Fund Research (HFR) and Eurekahedge. Both have an exhaustive database on the performance of quant funds and have created indices for easy tracking.
HFR has Emerging Market India Index, and Eurekahedge has India Long Short Equities Hedge Fund Index. They publish the performance of each month, going back several years.
A domestic solution is PMS AIF World that tracks the performance of PMS and AIF Cat III in India. This seems a better choice, but unfortunately, they do not publish month-wise performance, which makes analysis and comparison harder.
An overarching problem when benchmarking to other quant trading strategies is the lack of transparency in data collection. There are no regulations that mandate quant/hedge/AIF funds to declare their performance publically. It is entirely up to the fund manager.
The last approach is to not externally benchmark quant trading strategies at all. Instead, define returns target for the month/year and benchmark against it. You’re your own competition then.
Meanwhile, as a new player in the Indian capital market ecosystem, we currently benchmark ourselves against NIFTY50 with gap up/down. It’s understood by everyone and also tougher to beat.